Weather is an endlessly fascinating topic: It affects everybody and can literally be a matter of life and death.
Forecasting seems to many some kind of black art, but the basic principles are fairly simple: Collect a lot of observations, plug them into a set of theoretical models, and turn the crank repeatedly to advance the scenario in steps of a few minutes at a time. Eventually this produces predictions 12, 24, 48, 72, or however many hours in advance are desired.
In the modern era, this is all done by the National Weather Service (NWS), a federal government agency, operating with extreme transparency and posting almost all of their data publicly, in real-time. NWS publishes narrative summaries aimed at the general public, but they also publish a lot of fairly technical discussions about the judgment calls they make, such as which models best predict particular weather conditions, aspects of uncertainty and so on.
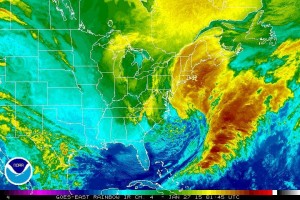
Although there are private weather forecasting agencies, none have anywhere close to the resources of NWS and therefore all rely on observations collected by NWS and on computer processing of models by NWS. Everyone is working from the same baseline data, and NWS is very good at what they do. A few decades ago, if someone wanted to go into the private weather forecasting business, the first thing they needed was a dedicated feed of NWS data that was intended to be continuously printed by a teletypewriter, but now all of that information and more is freely available on the internet from weather.gov and related sites. When NWS started putting data on the internet in the 1990s, it was mostly a minimally parsed dump of that old teletypewriter feed, and it was not very popular: I wrote a script to poll the NWS server every few minutes to see if any severe weather alerts had been posted, and to my astonishment their published logs showed that I was the 20th heaviest user in the world of that data feed. Within the last 15 years or so, it became realistically practical to dive into raw NWS data and compile what amounts to one’s own forecast.
Why would anyone want to do this? Typical media weather forecasts are very dumbed down, masking complexity that while inherent in the science is a challenge to convey. Does the ordinary consumer of a weather forecast want to be presented with charts and graphs showing probability distributions? There is a perceived risk that the public will lose confidence in a forecast if it is presented with too emphatic an admission of uncertainty, and this discourages the preservation of nuance.
Severe weather can involve snowstorms and hurricanes that most people understand are difficult to predict, but fairly ordinary weather presents hard-to-summarize problems, too. The forecast may say there is a chance of rain, but at what times of day is it likely to start and end? Is the probability distribution actually multimodal, where the most likely scenarios are a lot of rain or no rain at all?
A few years ago, I began for my own purposes compiling weather forecasts from NWS data. My main goal was to cut through the oversimplification of typical media weather forecasts, trying to understand the subtleties and uncertainties being discarded in the presentation. This proved useful, and I began developing ways to summarize the results over social media while preserving both narrative clarity and nuance. These became popular among my friends and followers.
Among my responsibilities at Motif is distribution manager: I co-ordinate the process of physically moving tens of thousands of copies of the newsprint magazine to a few thousand delivery points throughout Rhode Island and nearby Massachusetts. We depend upon a fleet of drivers to do this, and we work to a rigid schedule because of constraints from our printing contractor.
The turning point where my interest in weather and my role at Motif crossed paths was a year ago in March, when I realized a snowstorm was going to hit during distribution. In the early morning hours of Saturday, Mar 11, 2017, I sent e-mail to the core staff:
I don’t want to alarm anyone and it’s too early to predict with good reliability, but right now the most likely scenario for the weather is a major snowstorm from about 11pm Mon to 4am Wed… as a practical matter near-blizzard conditions are expected, especially at the peak of the storm 9am – 5pm Tue with wind gusts as high as 40MPH… the amount of liquid associated with this storm will have the potential to drop as much as 18 inches of snow on somebody.
Motif editor Emily Olson, then 8 ½ months pregnant, jokingly replied, “So my labor starts Tuesday afternoon. Got it.” (It didn’t.) The real problem was that, three days in advance of a near-blizzard, the broader news media were not even talking about it, so public awareness was consequently very low. Even our printing contractor was about to be caught by surprise. While weather forecasting seems an odd fit for a magazine that publishes twice per month, it made sense to disseminate the information I was already developing for internal purposes, so we posted it on social media. It proved a big hit.
I learned a lesson years earlier in what came to be known as the “December Debacle,” when allowing schools to open and then conduct early dismissal threw the state into chaos, with parents scrambling to leave work in time to meet their children and the students thrust out on buses at the peak of the storm, trapping everybody in traffic for many hours. As I wrote about 7:30pm on Thursday, Dec 13, 2007, while snow was still falling:
I knew from the weather forecast that things would be bad in Providence, but I honestly did not expect things to get as bad as they did. I have not seen snow-caused road conditions this bad since the legendary Great Blizzard of 1978.… the key mistake in 1978, as everyone realized in hindsight, was allowing schools and businesses to open normally, in defiance of the forecast… Today, we have much better technology to cope with these kinds of situations, but we don’t use it properly. Aside from the fact that weather forecasting itself is far more accurate further in advance [than in 1978], e-mail and cell phones make it possible to disseminate information very quickly in order to effect cancellation notices.
I had an evening event scheduled on the day of the storm, and I began watching the forecast a day and a half earlier, sending out e-mail updates and announcing more than 24 hours in advance that the event would be postponed if there was no change in the forecast. By the morning, about two hours before snow began, I officially postponed my event. As I wrote at the time:
What I did was not some sort of brilliant prophecy, but rather simply trusting the accuracy of the forecast. I had a lot of detailed information at my disposal, with the National Weather Service data showing that the snow was likely to start no earlier than 9am and no later than 1pm, detailed predictive information beyond the technology of 1978…. In the end, this snowstorm should be a learning experience. We need to use the improved technology of weather forecasting to make decisions about closing schools and businesses sufficiently far in advance to do any good, and we need to use the improved technology of communications to disseminate those decisions quickly and reliably.
The lesson I learned, personally, was that people often don’t believe weather forecasts: The “December Debacle” occurred because an excellent, high-quality forecast that accurately predicted the weather almost exactly correctly and sufficiently far in advance was simply ignored by schools and businesses as well as by individuals. Clearly, they had been conditioned not to trust weather forecasts, but why?
My conclusion is that the hiding of uncertainty and complexity diminishes trust in the forecast. If the scientific model predicts a 60% chance of rain, so the forecast is dumbed-down to “rain” but there is no actual rain, then people see this as a wrong forecast; in truth, the forecast should have explained that there was a 40% chance of no rain. It may be frustrating for the public to be told it is almost a toss-up whether there will be rain, but that’s what it is.
Snow accumulation is a noteworthy case because everyone wants to know how much we might get. In connection with a blizzard warning on Jan 4, 2018, I posted: “The median accumulation forecast for Providence is 10 in, with probabilities near 100% for at least 0.1 in, 98% for 1 in, 97% for 2 in, 93% for 4 in, 86% for 6 in, 75% for 8 in, 37% for 12 in, and near 0% for 18 in.” In my opinion, it is a disservice to dumb this down to the typical “8-12 inches.”
Regarding a weather event on Feb 7, 2018, I explicitly pointed out that while snow was likely we could get all rain: “The median accumulation forecast for Providence is 1 in, with probabilities 71% for at least 0.1 in, 55% for 1 in, 40% for 2 in, 7% for 4 in, and near 0% for 6 in. Note that this implies a 29% chance of no snow at all.” I described the most likely scenario, saying that precipitation “…is likely to begin after 9am as snow, change to sleet and freezing rain starting around 12pm, and complete a change to rain by 3pm… Precipitation may turn back to snow briefly before ending around 1am…” The typical presentation would dumb that down to “wintry mix.”
Such detailed narratives with actual numbers and times, in my opinion, enhance the credibility of the forecast by communicating uncertainty in a useful way. Removing complexity and nuance by dumbing down forecasts fools people into disbelieving weather forecasts that were highly accurate before being oversimplified for typical media presentation.
More Posts by The Author:
Existing is a Lot: A “F— Cancer” fundraiser
Severe Thunderstorm, Flash Flood Warnings: Reports of 1-inch diameter hail
Heavy Rain, High Winds, Flooding Wed–Thu: Advisory for wind, watches for inland and coastal flooding posted
River Flood Warnings in RI, MA, CT: Wed through Fri, streets may be impassable at times
Snow possible but unlikely Sat mid-day: Little accumulation likely
